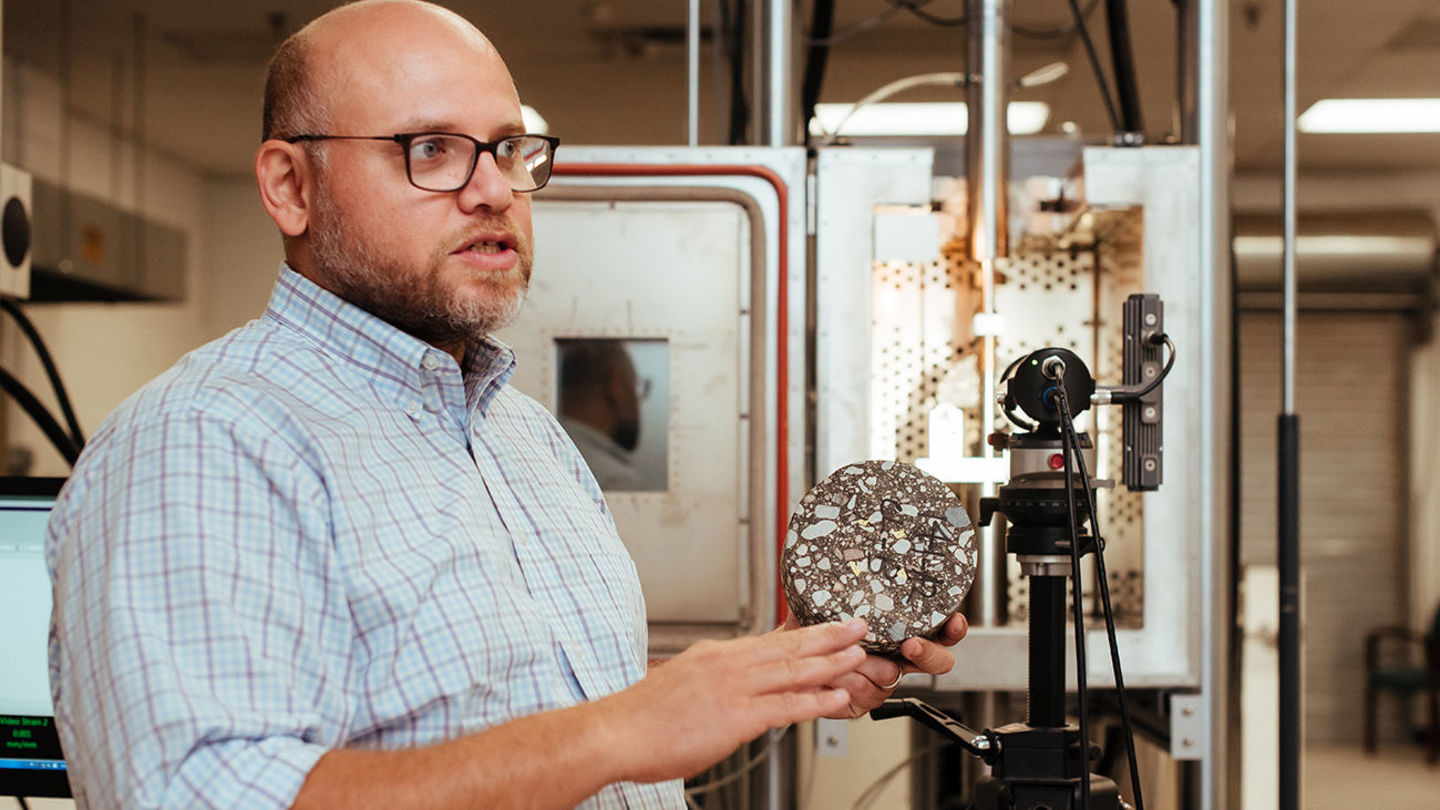
Americans take an average of 1.1 billion vehicle trips every day -- that's four trips for every person in the country. You can imagine the type of wear and tear that puts on the road surface. Actually...can you imagine?
Temple Engineering Assistant Professor of Civil Engineering Ahmed Faheem believes we can, using consistent data to more accurately measure the service conditions of pavement.
Working through a research award from the Wisconsin Department of Transportation, Dr. Faheem sought to use already-existing data on in-service performance on a project that could redefine how departments of transportation make decisions on road repairs.
"We're trying to understand what contributes to failures, successes and longevity and create models," Dr. Faheem said. "In this day and age, we have this data revolution. So much of this data on performance is available. We just need to connect it."
That data includes information pulled from on-site distress surveys and construction data from a previous project consisting of about 250 miles of Wisconsin's roadwork, from which his team created a geo-relational database. They use this database to study deterioration modeling using artificial intelligence and deep learning.
"When you're dealing with natural materials, in our case constitutes the majority component of asphalt paving, there is a high level of variability. Basically, the paving is a mix of about 95 percent aggregate and five percent asphalt that is blended in a big drum and driven to a site. Ideally, each batch is exactly the same as the one before, but that is not always the case," Dr. Faheem said. This variability opens the door to employ data science in understanding how production of asphalt mixes could influence performance.
As Dr. Faheem described, that variability can also be found within the very same stretch of road.
"Even some segments within the same road, built with the same material, the same structure design, same traffic, will see some segments fail before others. And until today, we are not sure why," he said.
According to Dr. Faheem, the project found that the geo-referencing technique allows for development of deterioration models that are unique to a specific pavement segment, unlike previously where segments were assumed to deteriorate at the same rate. In addition, the data-driven analysis directly connected climate and traffic loading patterns to specific failure types—a critical component for future road construction.
"The true breakthrough is in the analysis framework," Dr. Faheem added. "Though the findings will be unique to each state, the framework developed and tested in this research allows for better selection of materials and mixes using lab screening. Agencies will have the chance to learn from the past performance for better future roads."
Dr. Faheem noted he hopes the work will progress, with next steps including connecting artificial intelligence models to environmental sustainability targets for more accurate life cycle assessments, and measuring energy and material flow for more sustainable infrastructure.